Missed out on the Metadata Roadshow? Check out the session, "Metadata is the foundation for your Artificial Intelligence (AI) Strategy" to catch up. The session, led by John Horodyski and Misti Vogt, focuses on:
- Providing the data needed for effective machine learning.
- Powering tagging with artificial intelligence.
- Using AI tools like facial recognition and OCR to power your metadata.
Here are some of the highlights:
- 15% of attendees are already using A.I. with their DAM. 34% are not there yet, and 50% would like to be.
- Leveraging meaningful metadata in contextual ways. Using, categorizing, and accounting for data, provides the best chance for your return on investment with A.I. tools for digital asset management.
- Quality data equals smart data equals happy A.I. and ultimately good results.
- Data integrity is critical to A.I. machine learning, and trust and certainty that the data is accurate and usable is critical.
- Word metadata is the tactical application of the data to your content, and the management of that content to enable creation and discovery for the distribution and consumption of all the things you're trying to manage.
- The best way for A.I. to learn is by doing, working with good data. Make sure you have good quality data in your digital asset management system.
- Most applications of artificial intelligence in DAM relate to metadata.
- The A.I. we're focused on today is designed to augment human intelligence in the digital asset management process, not replace it.
- Using third party solutions within your DAM system is the best way to get more reliable predictions. This is because A.I. learns and refines its results by repeated exposure to data. By consuming massive volumes of data third party solutions can return more accurate results than a custom-built AI used in only one DAM system.
- Using a combination of different systems: your DAM system, A.I. and you (the human element) creates a robust, well rounded keyword framework for your assets.
- A.I. in tandem with your digital asset management platform can do a pretty good job of setting up foundational key words. People are still important to augment that intelligence - especially for sentiment, which A.I. isn't as good at yet. People also augment by specifying nuances that A.I. may not see. An example is a body of water - it could be a lake, a river, or an ocean. A.I. would have a hard time differentiating, but a human can do so in seconds.
- There are factors that even humans can get wrong or simply shouldn't guess - such as race, gender, or other sensitive labels. This should be factored into building your A.I., for example by building a block list to accommodate use cases for labels that should be systematically ignored.
- It is also important to prevent the A.I. from generating its own unvetted terms. You will need to add boundaries and rules regarding new keywords, for example 85% match from at least two A.I. providers. You can set parameters to add all words, ignore all new words, or find and flag all new words to be vetted by a human for inclusion or exclusion.
- By leveraging A.I. to set the foundation of keywords, you can save massive amounts of time and money versus only using humans to perform all digital asset management keywording tasks.
- A.I. is also very effective at streamlining image identification using facial recognition. This can save organizations thousands of dollars in digital asset management workload. In one example, the cost was 3 times less using A.I. than for manually tagging faces.
- For processing and captioning video, A.I. is also a great ally. Natural language processing enables you to quickly caption video assets so they are searchable, and connecting the captions to the exact section of video makes it easy to jump to that section of script and video. Again, it's important for this functionality to be editable by permissioned users in order to correct any errors made by the A.I.
Ultimately, artificial intelligence is an incredibly valuable digital asset management tool for organizations to take advantage of. However, you need to have the right system and people in place to support it.
To learn more about how Orange Logic can help you with your DAM needs, schedule a call today!
Bring it all together with an intuitive, customizable DAM platform.
Cortex is an Enterprise Digital Asset Management Platform built to grow with your business.
- 130+ custom tools
- Tailored dashboards for every user
- Unlimited digital asset storage
Request Demo
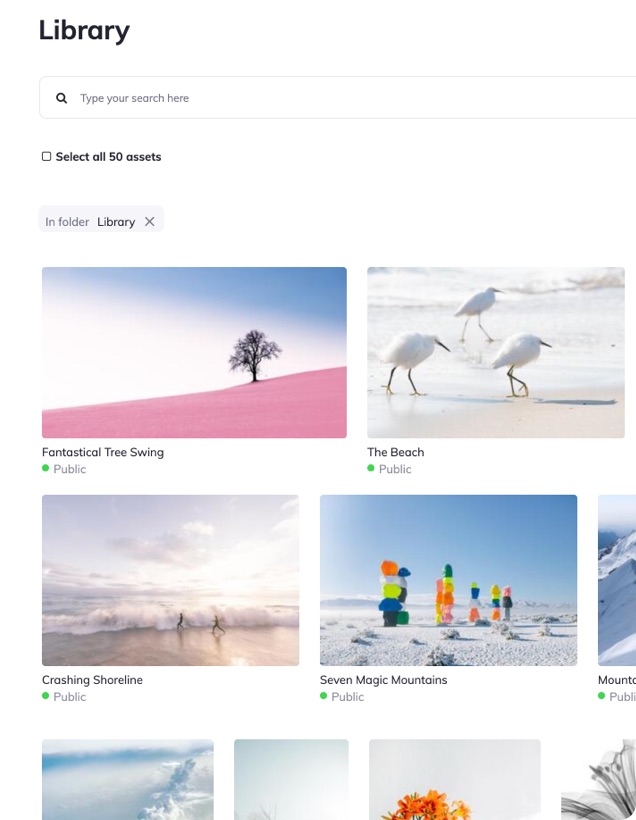
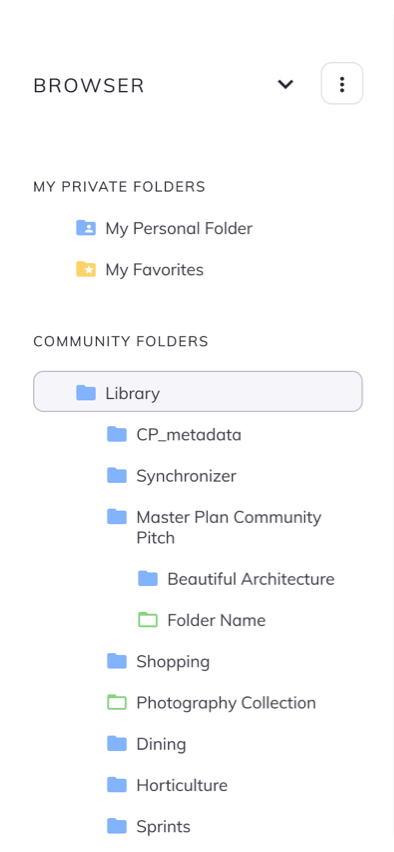
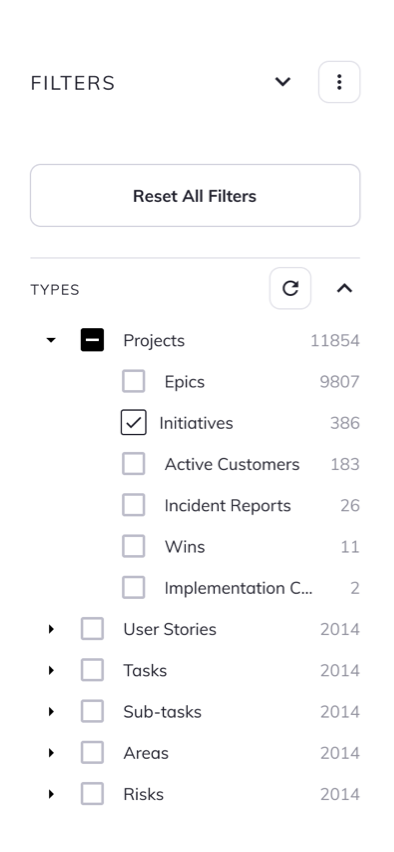